Overview
The Initiative's interdisciplinary strategy is one that has been central to UC Santa Barbara’s success. Our synergistic approach brings together computational scientists studying the human mind with computer scientists and engineers to create new ways of looking at fundamental problems in science, engineering, and humanities that are highly relevant to modern society. The Initiative will pave an innovative and transformational path forward by focusing on the development of the next generation of machine intelligence and increasing our understanding of the underlying brain computations that mediate the complex cognitive abilities that make humans unique. The Mellichamp Faculty Cluster in Mind and Machine Intelligence is endowed by Duncan and Suzanne Mellichamp who have generously supported several timely initiatives that have helped drive the impressive growth of UC Santa Barbara over the past 50+ years. Duncan is Emeritus Professor of Chemical Engineering and Suzanne is a retired educator of the Santa Barbara Public Schools.
Leadership
The Initiative's goals center our research around the following aims
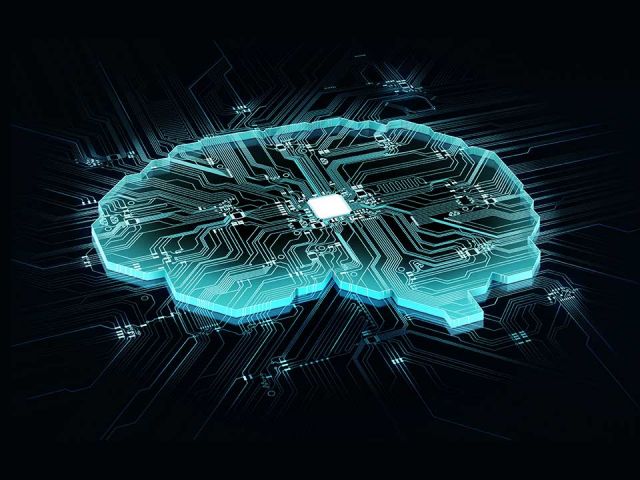
Improve the capabilities of machine intelligence
Machines have surpassed human capabilities in increasingly complex arenas, an accelerating trend that can be clearly seen for games such as checkers (1990), chess (1997), Jeopardy (2011), Atari (2013), and GO (2016). Yet humans are still superior at many natural tasks for which our brains evolved to perform in the uncertain complexity of the real-world that span the domains of perception, language, learning, and decision-making. A deep understanding of the computational differences between humans and machines will allow us to improve intelligent machines in all of these domains. What are the differences between how deep neural networks and humans see? Can machines achieve human-level linguistic capabilities by understanding how humans incorporate context, inference, and common sense to reason beyond the literal text? How can babies learn some complex concepts from just a few examples while machines require exorbitantly large training data sets? Insights gained from exploration of these cognitive distinctions could improve the ability of machines to actively learn from experiences, including those that might not be directly related to the immediate goals, underpinning the robustness and adaptability that AI systems require to function in the real world.
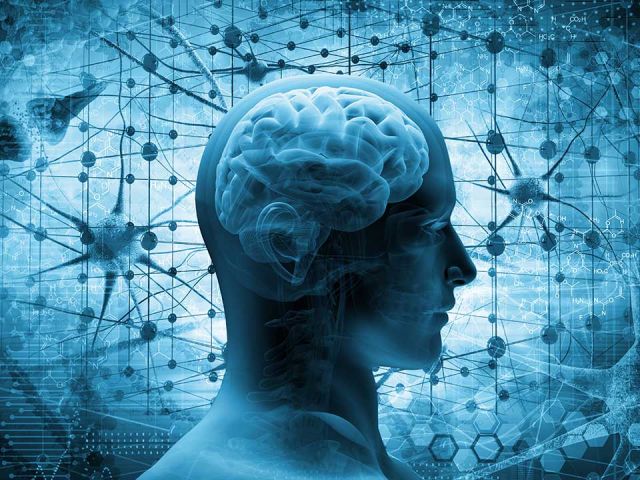
Advance understanding of the cognitive functions of the human brain and mind
Although there is a long history of computational modeling in the perceptual and cognitive sciences, most of these models use simplified laboratory tasks and parameter spaces that presume knowledge of the statistical distributions of the inputs. The models often fail at complex natural tasks such as finding a person in a cluttered scene, inferring a person's social intent, understanding naturally occurring speech, learning complex categories that generalize across domains, or making real-world economic decisions. The use of AI as a research tool will inform cognitive scientists, behavioral economists, and neuroscientists about the computations that are critical, and possibly unique, to human cognition.
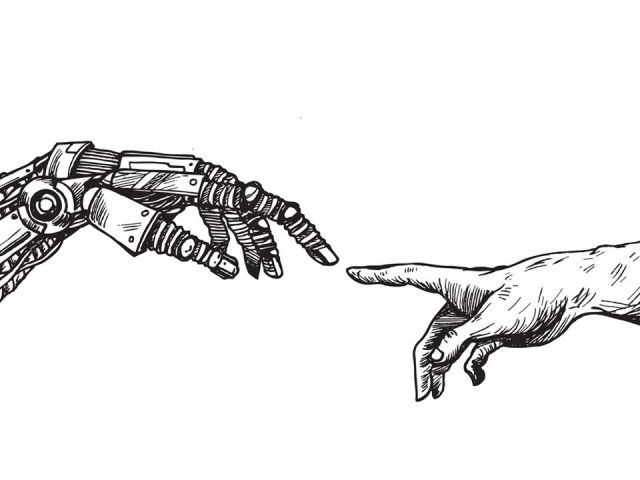
Understand and optimize the integration of humans and machines
Spanning fields from radiology to security, and applications from natural disaster management and defense to insurance and consumer decisions, a growing number of life-critical societal decisions are being made by mixed teams composed of both humans and machines. For example, breast screening machines that identify regions in 3D image volumes that might contain cancer and then focus the radiologist's visual scrutiny on that subset of image regions. Or AI that assists consumers deciding among a large number of complex health insurance plans by selecting only the best possible options. Yet our understanding of how cognition operates in human-machine mixed team decision-making is still in its infancy. The Initiative will contribute to the rising field of human/robot engineering by identifying the different strengths and limitations of humans and machines and how to best integrate them for the benefit of humans.
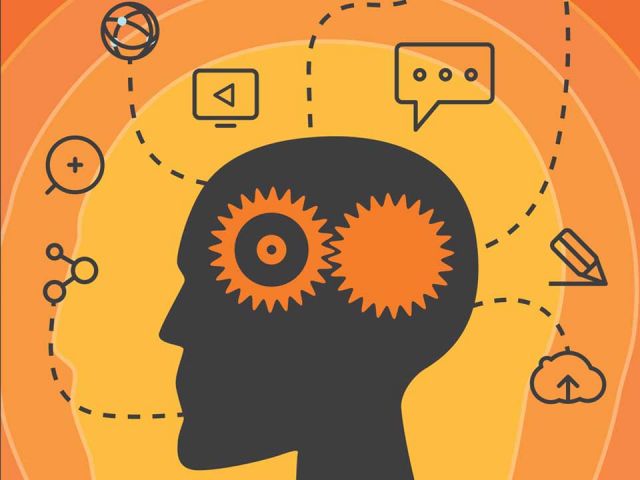
Assess the impact of machine intelligence on normal human cognitive function
The increased use of machines and technology by humans from an early age can have profound effects on brain function and cognitive development spanning the domains of attention, learning, spatial navigation, memory, and decision making. As intelligent devices become ubiquitous and increasingly necessary in human societies, it becomes imperative that we gain a deep understanding of the effects of technology on human cognitive function through rigorous studies that integrate behavioral paradigms, neuroscience tools, and computational modeling.