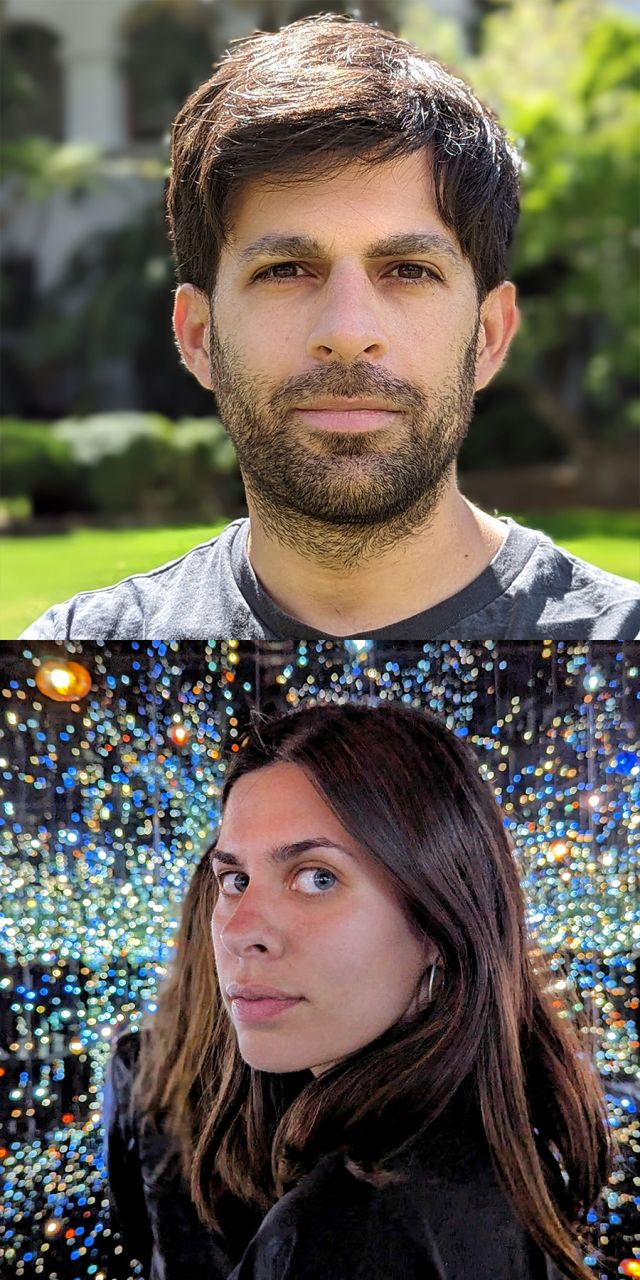
UCSB students Iason Paterakis and Nefeli Manoudaki, Winner of the Mellichamp Mind and Machine Intelligence's 2024 AI/Human Visual Art Creativity Contest
“Osmosis”_ XR urban fluctuations using Generative AI by Iason Paterakis and Nefeli Manoudaki
Affiliation: transLAB, Media Arts and Technology Program, UCSB. Director: Marcos Novak
Artist Statement
High-tech interactive façades, projection-mapped buildings, and LED walls shape the future of augmented cities. Media-driven surfaces are found in Times Square, Shibuya Crossing, COEX K-Pop Square, Fremont Street Experience, and Piccadilly Circus, with the "Sphere" in Las Vegas standing as a prime example.
"Osmosis" is a series of large-scale, real-time urban installations related to transmodal design in Extended Reality (XR) using generative AI. We explore the potential advancements in immersive space design and generative Artificial Intelligence (AI) by demonstrating a methodology for creating AI-driven immersive urban environments. This approach is inspired by the biological process of osmosis, where solvent molecules traverse a semipermeable membrane along a concentration gradient. We translate this concept into a methodological framework that treats AI-generated visual content as the 'solute' and the built environment as the 'solvent,' with the projection surface acting as a semipermeable membrane. By algorithmically modulating the 'osmotic pressure' of visual elements, we facilitate a controlled diffusion of digital information into the architectural space. This enables a gradient-driven flow of AI-generated content, allowing for the emergence of hybrid spaces that challenge traditional architectural boundaries.
Three generative projection mapping installations at the Santa Barbara Center for Arts, Sciences, and Technology (SBCAST) serve as case studies. Each iteration experimented with generative text-to-video, image-to-video, and video-to-video AI models, integrating software like Blender and TouchDesigner for realtime graphics. The primary AI models utilized were Diffusion Models, specifically StreamDiffusion, RunwayML’s Gen-1, and Stability AI’s Stable Diffusion. The proposed pipeline expands the method of using natural language prompts to immersive urban spaces, allowing users to modify the environment interactively. This framework serves as a foundation for generating architectural content using Generative AI and Extended Reality mediums, creating a seamless blend between physical and virtual realms.
Initially, Blender was utilized for procedural 3D modeling of building volumes, integrating effects through geometry nodes, and Python interface. Fluid simulations and particle systems were incorporated within virtual cubes to simulate real-time dynamics. AI models like Stable Diffusion, MidJourney, and DALL·E generated images depicting abstract biological formations, which were then used as inputs for further AI video generation processes. For generative video, RunwayML Gen-1 was initially used to generate video outputs, while SteamDiffusion later allowed for real-time manipulation of AI-generated content within TouchDesigner (Stream Diffusion, DostSimulate TD node). Finally, TouchDesigner facilitated the overlay of multiple layers of Perlin noise and blending effects, enhancing interactivity and integration of videos with real-time layers. The technical setup included distributed computing, ensuring smooth execution of real-time generative processes. One machine handled projection mapping via MadMapper, while another streamed real-time AI video content using Network Device Interface (NDI) over IP, with communication managed through an Open Sound Control (OSC) network for data exchange. This setup allows additional computers to be added for audio and visual generation, facilitating collaboration among multiple artists and engineers and using the building for live experimentation.
This fusion introduces complex forms and real-time data-driven collaboration, creating a harmonious ecosystem where urban space, AI-driven data, and its inhabitants can operate cohesively. Future research should consider incorporating additional sensory inputs, such as haptic and olfactory interfaces, and developing neural networks trained on observer preferences to inform Diffusion AI models about visual complexity, color, patterns, and other characteristics.
Acknowledgements: Ryan Millett PhD student, MAT, UCSB (generative sound design), Sabina Hyoju Ahn
PhD candidate, MAT, UCSB (bio-synthesizer).
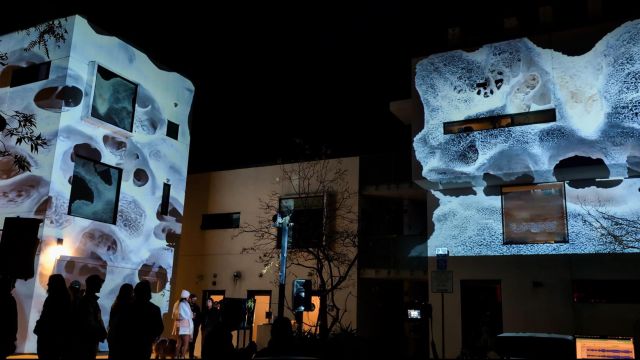
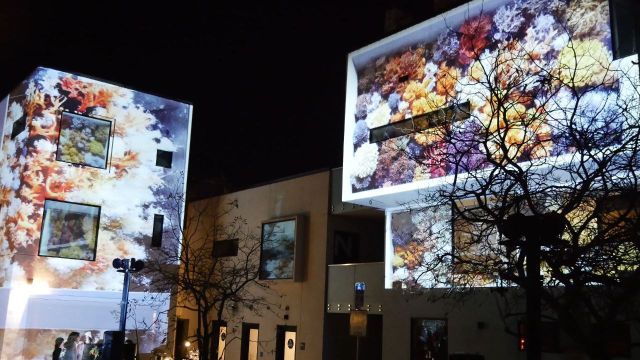
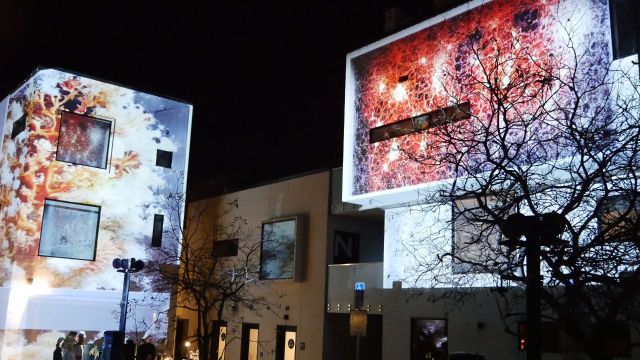
