Mellichamp Initiative in Mind & Machine Intelligence Summit 2023
Theme: AI and Decision Making

Overview
AI’s ability to see, predict, communicate, and persuade humans is increasing every month. Sometimes, AI potentiates humans, other times, it hinders humans. AI can show behaviors and creations that are unanticipated by its designers. Some, enlighten us and others alarm us deeply. As AI becomes an increasing protagonist in our lives from driving and healthcare to justice and everyday decisions, we must gain a better understanding of the intricacies of AI, the human mind, and how humans and AI learn from each other to interact better.
The 2023 Mellichamp Mind and Machine Intelligence Annual Summit brings together leaders in AI, Human/AI Interaction, Psychology, and Economics to present the latest on AI and Decision Making and debate timely questions. The meeting will be held over two half days (April 13th and 14th, 2023).
Agenda
- April 13 (Session 1) AI and Human Cognition
-
AI and Human Cognition
8:30am Krzysztof Gajos
Harvard University
"Human Cognitive (Dis)Engagement during AI-Assisted Decision-Making"9:00am
Danny Oppenheimer
Carnegie Mellon University
"Decision Science in the Age of Augmented Cognition"9:30am Misha Sra
University of California, Santa Barbara
"Human-AI Integration"10:00am Panel Discussion
Moderator: Miguel Eckstein, University of California, Santa Barbara - April 13 (Session 2) AI, Welfare, Equity, and Justice
-
AI, Welfare, Equity, and Justice
11:00am Elliott Ash
ETH Zurich
"AI for Governance: Detecting Corrupt Mayors and Biased Judges"11:30am
Emma Pierson
Cornell University
"Using Machine Learning to Increase Equity in Healthcare and Public Health"12:00pm Daniel Bjorkegren
Brown University
"Welfare Sensitive Machine Learning"12:30pm Panel Discussion
Moderator: Heather Royer, University of California, Santa Barbara - April 13 (Session 3) AI, Human Preferences and Beliefs
-
AI, Human Preferences and Beliefs
2:30pm Berkeley J Dietvorst
University of Chicago
"Aligning Algorithm's with People's Prediction Preferences'"3:00pm
Daniel Martin
University of California, Santa Barbara
"Labeling and Training with Elicited Beliefs'"3:30pm Panel Discussion
Moderators: Antony Millner, University of California, Santa Barbara - April 13, Keynote Lecture: "Algorithms and People"
-
4:00pm Sendhil Mullainathan
University of Chicago
"Algorithms and People"Moderator: Kelsey Jack, UC Santa Barbara Bren School of Environmental Science and Management - April 14 (Session 1) AI-Human Decisions, Trust, and Theory of Mind
-
AI-Human Decisions, Trust, and Theory of Mind 8:30am Hima Lakkaraju
Harvard Business School
"Explainable Machine Learning: Challenges and Opportunities"9:00am
Mark Steyvers
University of California, Irvine
"Mental Models in Human-AI Collaboration"9:30am S. Shyam Sundar
Penn State
"Responsible AI: Enabling User Calibration of Trust with Interactive Interfaces"10:00am Panel Discussion
Moderator: William Wang, University of California, Santa Barbara - April 14 (Session 2) AI-Human Collaboration
-
AI-Human Collaboration 11:00am Ming Yin
Purdue University
"Towards a Science of Human-AI Decision Making: Empirical Understandings, Computational Models, and Intervention Designs"11:30am
Gagan Bansal
Microsoft Research
"Understanding and Improving AI-Assisted Programming"12:00pm Ambuj Singh
University of California, Santa Barbara
"Explaining Group Decision Processes Under Risk"12:30pm Panel Discussion
Moderator: Lei Li, University of California, Santa Barbara
AI, Welfare, Equity, and Justice
AI, Human Preferences and Beliefs
Keynote Lecture: "Algorithms and People"
AI-Human Decisions, Trust, and Theory of Mind
Organizers
- Miguel Eckstein Psychological and Brain Sciences
- William Wang Computer Science
- Mary Hegarty Psychological and Brain Sciences
- Ruimeng Hu Mathematics and Statistics and Applied Probability
- Lei Li Computer Science
- Daniel Martin Economics
- Mark Steyvers Cognitive Sciences (UCI)
- Joe Walther Communication
Dates & Location
April 13-14, 2023
Henley Hall 1010
UC Santa Barbara
Talk Abstracts and Speaker Bios
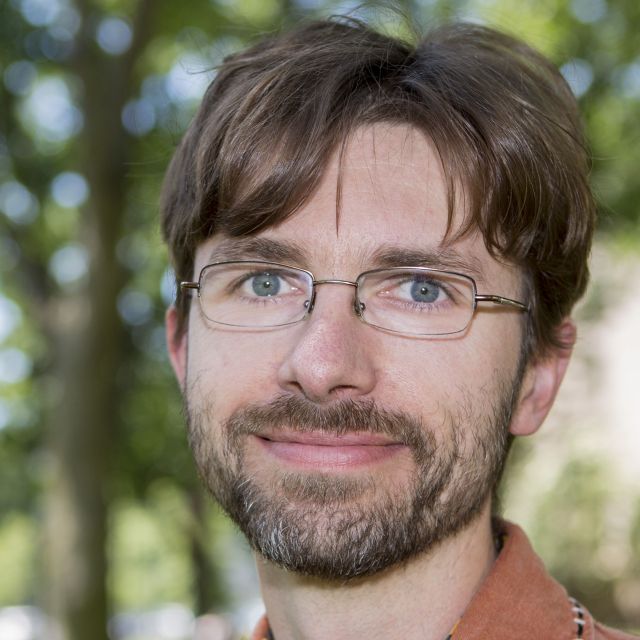
Krzysztof Gajos
Gordon McKay Professor of Computer Science
Harvard Paulson School of Engineering and Applied Sciences
"Human Cognitive (Dis)Engagement during AI-Assisted Decision-Making"
Abstract: People supported by AI-powered decision support tools were expected to make better decisions than either people or AI systems on their own. In practice, this is almost never the case. Based on the evidence that people over-rely on the AI recommendations, we posit that in most settings people do not cognitively engage with the AI-provided information (recommendations or explanations) leading to the poorer-than-expected outcomes. This problem has gone largely undiagnosed because the prevalent methods for evaluating human-AI interaction innovations rely on proxy tasks which, we demonstrate, produce misleading results. We then demonstrate that cognitive forcing (an intervention intended to push people toward more analytical processing of information) can reduce human over-reliance on the AI but possibly without addressing the root cause. Finally, we introduce incidental learning as an indirect but objective indicator of cognitive engagement. Using this as our dependent measure, we provide some of the most direct evidence to date that people really do not engage cognitively with the AI-provided information in the standard explainable AI settings, even when cognitive forcing is applied. Instead, we observed both high quality decisions and strong evidence of cognitive engagement when people were provided by an AI with informative explanations but no decision recommendations. These results provide early evidence that in some settings a better human-AI interaction paradigm may be to use the power of AI to construct actionable syntheses of the information to aid people in making their own well-informed decisions rather than recommending the ready-made decisions to them.
Bio: Krzysztof Gajos is a Gordon McKay professor of Computer Science at the Harvard Paulson School of Engineering and Applied Sciences. Krzysztof’s current interests include 1. Principles and applications of intelligent interactive systems; 2. Tools and methods for behavioral research at scale (e.g., LabintheWild.org); and 3. Design for equity and social justice. He has also made contributions in the areas of accessible computing, creativity support tools, social computing, and health informatics.
.
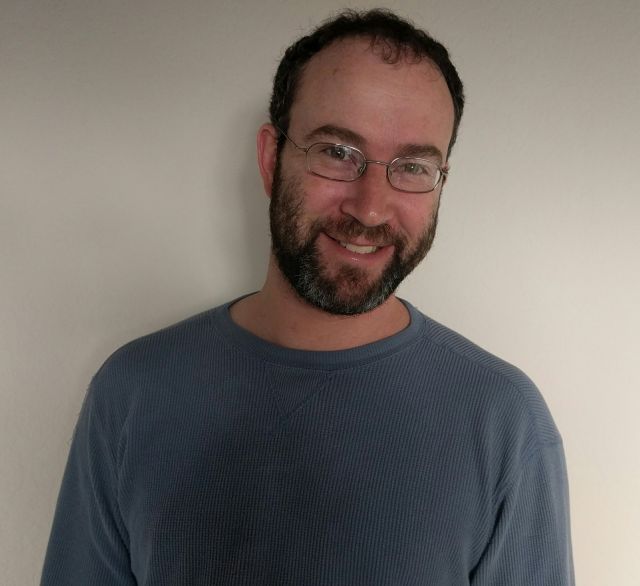
Danny Oppenheimer
Professor of Psychology and Decision Sciences
Carnegie Mellon University
"Decision Science in the Age of Augmented Cognition"
Abstract: While most psychologists focus on thinking that occurs in the brain, most would also acknowledge that cognition is not exclusively accomplished by the brain, but by an interaction between brains, tools, and environments. According to the "extended mind" perspective, cognitive processes are often offloaded to various technologies, freeing our limited cognitive resources for more complex thought. Extending cognition to our environment is not new, however with recent advances in artificial intelligence and machine learning, cognition enhancing devices are being developed at unprecedented rates. Using augmenting technologies does not merely improve our thinking, but in many ways can qualitatively change the nature of how we think. Different media lead us to ask different questions, remember (or forget) different information, attend to different details, and interact with other people in different ways. These types of thinking aren't inherently better or worse, but they may be better or worse for facilitating specific goals, change our decisions, and impact the effectiveness of policy interventions. In this talk, I will discuss why it is important for decision scientists to extend our frameworks to account for extended cognition, and highlight some recent research from my own lab that explores how the use of technology can impactfully affect how we think and behave.
Bio: Danny Oppenheimer is a professor at Carnegie Mellon appointed in Psychology and Decision Sciences who studies judgment, decision making, metacognition, learning, and reasoning, and applies his findings to such diverse domains as charitable giving, education, electoral outcomes, technology, and how to trick students into buying him ice cream. He has won awards for research, teaching, and humor, the latter of which is particularly inexplicable given his penchant for terrible puns.
.
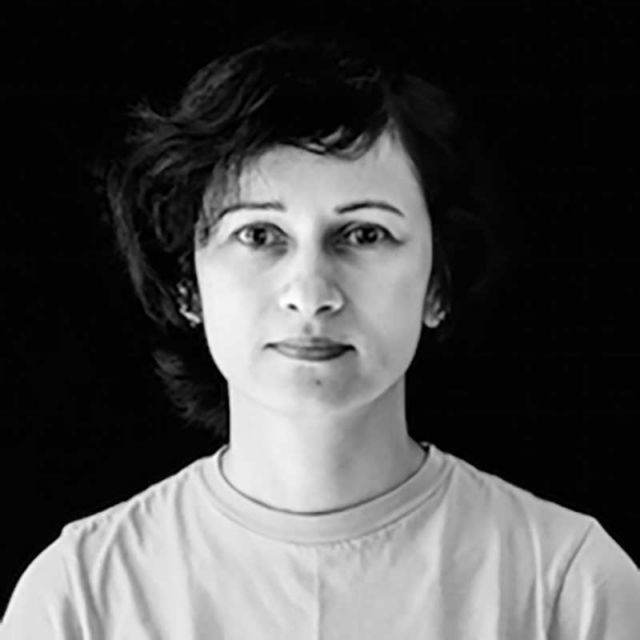
Misha Sra
John and Eileen Gerngross Assistant Professor of Computer Science
University of California, Santa Barbara
"Human-AI Integration"
Abstract:The biological evolution of early humans was paralleled by their use of materials to build tools. Tools allowed humans to become successful at reliably getting food and surviving predators. In fact, human prehistory can be divided into periods named after the material predominantly used to build tools during that period. Stone was used first for about three million years, followed by bronze and iron. These materials fundamentally changed civilizations. The advent of new tools led to numerous advancements including better production of food, improved healthcare and longevity, and enhanced quality of life.
If we think of AI as the material of our times, the big question is - what tools will we build with AI that will improve our lives? A lot of the current effort is going into improving the material, with new and impressive algorithms and models being released almost daily. However, not much work has yet gone into using this new material to build tools for solving real world needs of people.
In this talk I will present research on the design of AI-based tools with particular focus on therapy and accessibility.
Bio:Misha Sra is the John and Eileen Gerngross Assistant Professor and directs the Human-AI Integration Lab in the Computer Science department at UCSB. Misha received her PhD at the MIT Media Lab in 2018. She has published at the most selective HCI and VR venues such as CHI, UIST, VRST, and DIS where she received multiple best paper awards and honorable mentions. From 2014-2015, she was a Robert Wood Johnson Foundation wellbeing research fellow at the Media Lab. In spring 2016, she received the Silver Award in the annual Edison Awards Global Competition that honors excellence in human-centered design and innovation. MIT selected her as an EECS Rising Star in 2018. Her research has received extensive media coverage from leading media outlets (e.g., from Engadget, UploadVR, MIT Tech Review and Forbes India) and has drawn the attention of industry research, such as Samsung and Unity 3D.
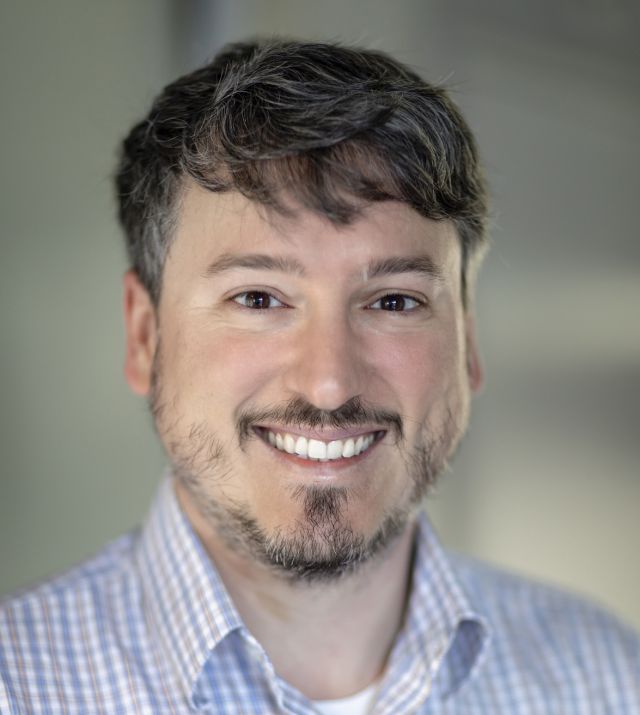
Elliott Ash
Assistant Professor Law, Economics, and Data Science
ETH Zurich
"AI for Governance: Detecting Corrupt Mayors and Biased Judges"
Abstract: Can AI tools support better governance? This talk discusses two research applications exploring this question.
First, In the context of Brazilian municipalities, 2001-2012, we have access to detailed accounts of local budgets and audit data on the associated fiscal corruption. Using the budget variables as predictors, we teach an AI classifier to predict the presence of corruption in held-out test data. We show how the predictions can be used to support policies toward corruption; relative to the status quo policy of random audits, a targeted policy guided by the machine predictions could detect more than twice as many corrupt municipalities for the same audit rate.
Second, using data on criminal cases in the U.S. state of Wisconsin, we build a recidivism prediction model that accurately ranks defendants by their probability of repeat offending. We use this model to help analyze potential biases by judges along identity margins such as race, age, and gender. There are large disparities in judge sentencing rates across groups that are not explained by differences in recidivism risk, but they cannot be explained by in-group bias (judge favoritism for the same group) either. Further, we find a racial in-group difference in the response to a recidivism risk: judges are more lenient for same-race defendants who are low risk but harsher for same-race defendants who are high risk, consistent with a shared in-group giving judges a more precise signal on the riskiness of defendants.
Bio: Elliott Ash is building a robot judge. As a professor at ETH Zurich's Center for Law & Economics, Elliott investigates the workings of law and policy through the lens of data science. Using natural language processing to sift through legal texts, and with natural experiments to get at causation, this research produces evidence to better understand how legal decisions are made. In the future, this work will provide a framework to support fairer decisions. Prior to joining ETH, Elliott held academic positions at University of Warwick and Princeton University, and before that earned a Ph.D. in economics and a J.D. from Columbia University.
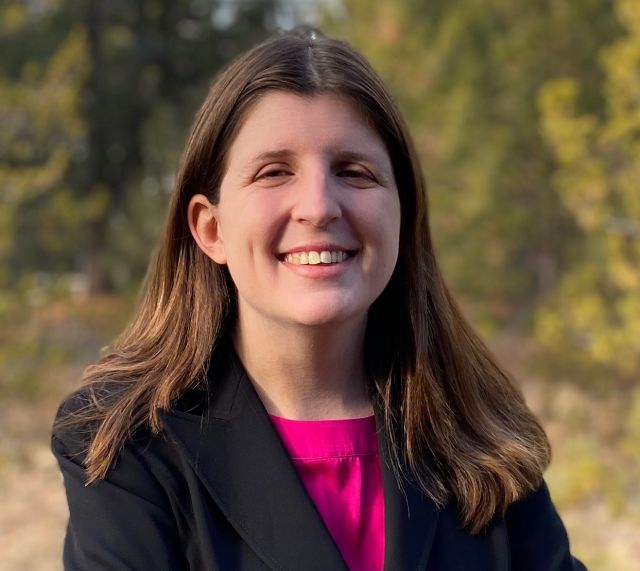
Emma Pierson
Assistant Professor of Computer Science
Cornell Tech
"Using Machine Learning to Increase Equity in Healthcare and Public Health"
Abstract: Using machine learning to increase equity in healthcare and public health. Abstract: Our society remains profoundly unequal. This talk discusses how data science and machine learning can be used to combat inequality in health care and public health by presenting several vignettes about policing and cancer risk prediction.
Bio: Emma Pierson is an assistant professor of computer science at the Jacobs Technion-Cornell Institute at Cornell Tech and the Technion, and a computer science field member at Cornell University. She holds a secondary joint appointment as an Assistant Professor of Population Health Sciences at Weill Cornell Medical College. She develops data science and machine learning methods to study inequality and healthcare. Her work has been recognized by best paper, poster, and talk awards, an NSF CAREER award, a Rhodes Scholarship, Hertz Fellowship, Rising Star in EECS, MIT Technology Review 35 Innovators Under 35, and Forbes 30 Under 30 in Science. Her research has been published at venues including ICML, KDD, WWW, Nature, and Nature Medicine, and she has also written for The New York Times, FiveThirtyEight, Wired, and various other publications.

Daniel Bjorkegren
Assistant Professor of Economics
Brown University
"Welfare Sensitive Machine Learning"
Abstract: How can machine learning systems account for society's preferences? We explore training ML methods to balance multiple objectives and preview an application to 'welfare' credit scores based on a digital credit experiment in Nigeria. We also develop a method that aims to close the loop between societal debate and algorithm implementation. We will demonstrate this by auditing the preferences implicit in the algorithmic targeting of Mexico's PROGRESA antipoverty program.
Bio: Daniel Björkegren is an Assistant Professor of Economics at Brown University. He works to make machine learning more humane, and apply it to problems in developing countries. His method to evaluate creditworthiness based on mobile phone usage was featured on NPR. He holds a Ph.D. in Economics and a Master’s in Public Policy from Harvard University.
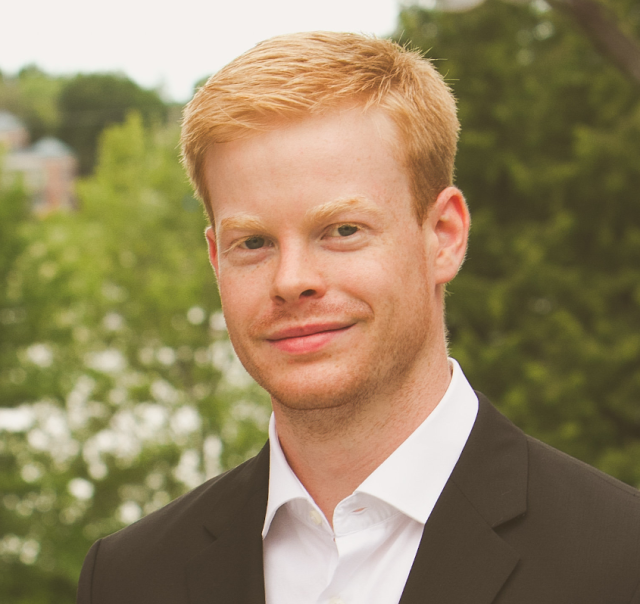
Berkeley J. Dietvorst
Associate Professor of Marketing
University of Chicago Booth School of Business
"Aligning Algorithm's with People's Prediction Preferences"
Abstract: Many companies promote predictive algorithms to consumers in the form of recommendation systems, navigations apps, robo-advisors, and many others. However, little is known about consumers’ preferences for predictions. In this work, I explore the goals that consumers adopt when making predictions, and test whether they are more likely to use algorithms built to accomplish those same goals. I find that most people who are asked to make a prediction adopt the goal of maximizing the frequency of perfect predictions. This contrasts with the goals of many popular algorithms, which are often built with objectives like minimizing squared error, minimizing average absolute error, or maximizing a likelihood function. Further, I find that people making incentivized predictions prefer algorithms built to maximize the frequency of perfect predictions to those built to minimize some function of error. Finally, I find that people are more likely to adopt algorithms built to maximize perfect predictions when choosing whether to use an algorithm or their own judgment for an incentivized prediction.
Bio: Berkeley Dietvorst’s research focuses on understanding how consumers and managers make judgments and decisions, and how to improve them. His main focus has been investigating when and why forecasters choose not to use algorithms that outperform human forecasters, and explores prescriptions that increase consumers’ and managers’ willingness to use algorithms.
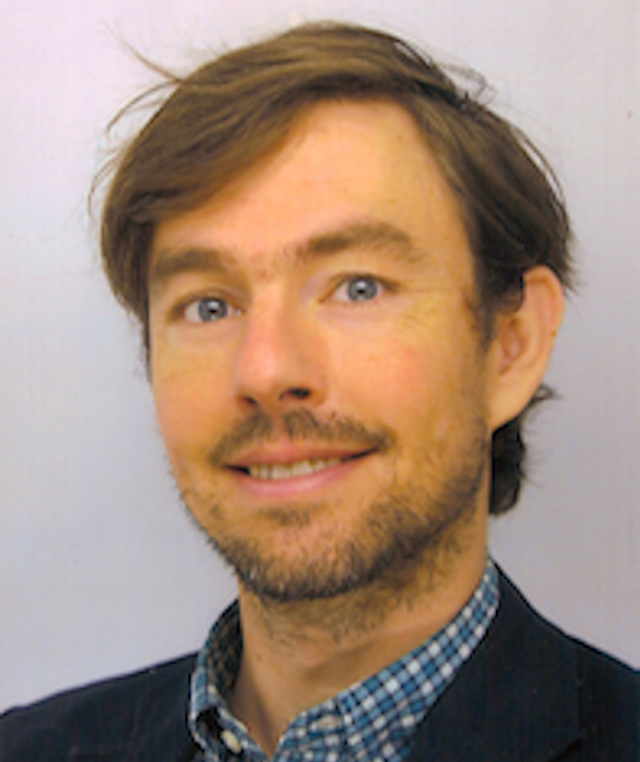
Daniel Martin
Assistant Professor of Economics
University of California, Santa Barbara
"Labeling and Training with Elicited Beliefs"
Abstract: We introduce the use of incentive-compatible belief elicitation for labeling data and training machine learning models. Eliciting beliefs truthfully through proper scoring rules is now standard in experiments and surveys, but has not yet been applied to labeling or training. We conduct an online experiment in which participants were incentivized to truthfully report their belief that a white blood cell was cancerous for a series of cell images and propose three methods for labeling each image based on participant reports. We evaluate these method by training a convolutional neural net on the labels they generate and find that they outperform standard labeling methods in terms of both accuracy and calibration.
Bio: Daniel Martin is the Wilcox Family Chair in Entrepreneurial Economics and an Associate Professor in the Economics Department at the University of California, Santa Barbara. He is a behavioral and experimental economist who studies how information is processed (attention and perception) and how information is communicated (information disclosure). His research has appeared in the top journals of the American Economic Association, Royal Economic Society, and European Economic Association. Before receiving a PhD in Economics from New York University, he was the co-founder of a small business, which is now one of the leading providers of IT services to small and medium-sized businesses in the Carolinas. Professor Martin teaches upper-division undergraduate courses on entrepreneurship and a PhD class on attention and perception.
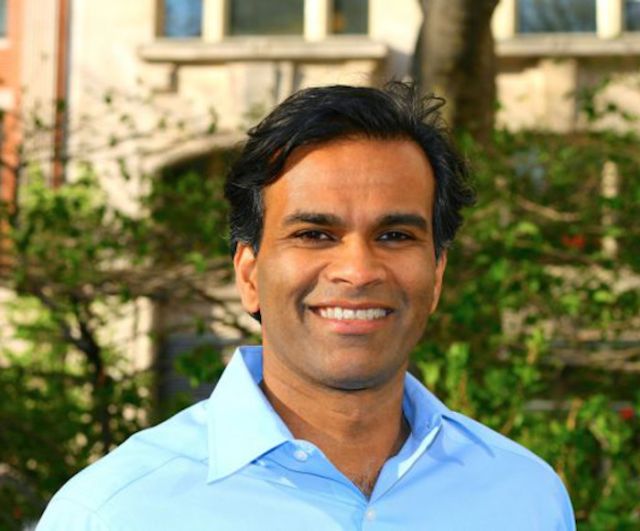
Sendhil Mullainathan
Roman Family University Professor of Computation and Behavioral Science
University of Chicago Booth School of Business
"Algorithms and People"
Abstract: In this talk, I walk the same road in two directions. First, I argue "We can use psychology to improve how we design algorithms." By this, I do not mean using insights about the brain to motivate the architecture of deep learning algorithms. Instead, I focus on how behavioral science findings (such as from the JDM literature) could improve widely used algorithms such as recommender systems. Second I argue "We can use algorithms to improve how we understand people." In particular, I describe computational tools that use machine learning algorithms to produce new theoretical insights about people. I conclude with the question of how we can create more traffic in both directions from each of these fields to the other.
Bio: Sendhil Mullainathan is the Roman Family University Professor of Computation and Behavioral Science at Chicago Booth, where he is also the inaugural Faculty Director of the Center for Applied Artificial Intelligence. His latest research is on computational medicine—applying machine learning and other data science tools to produce biomedical insights. In past work he has combined insights from behavioral science with empirical methods—experiments, causal inference tools, and machine learning—to study social problems such as discrimination and poverty. He currently teaches a course on Artificial Intelligence. Outside of research, he co-founded a non-profit to apply behavioral science (ideas42), a center to promote the use of randomized control trials in development (the Abdul Latif Jameel Poverty Action Lab), has worked in government in various roles, and currently serves on the board of the MacArthur Foundation board. He is also a regular contributor to the New York Times.
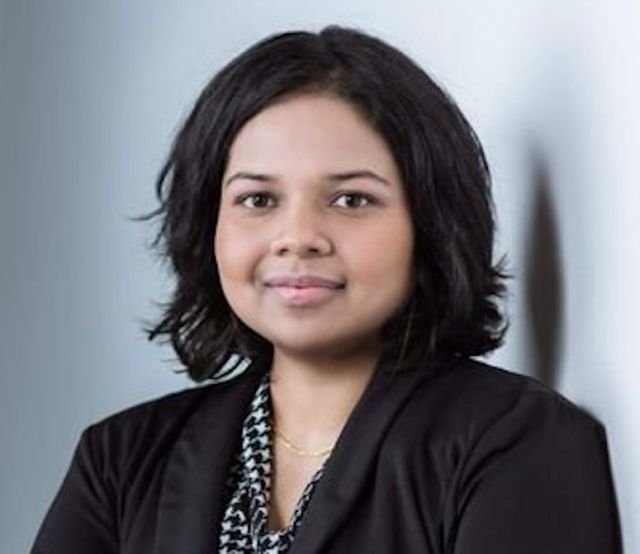
Hima Lakkaraju
Assistant Professor of Business Administration and Computer Science
Harvard University
"Explainable Machine Learning: Challenges and Opportunities"
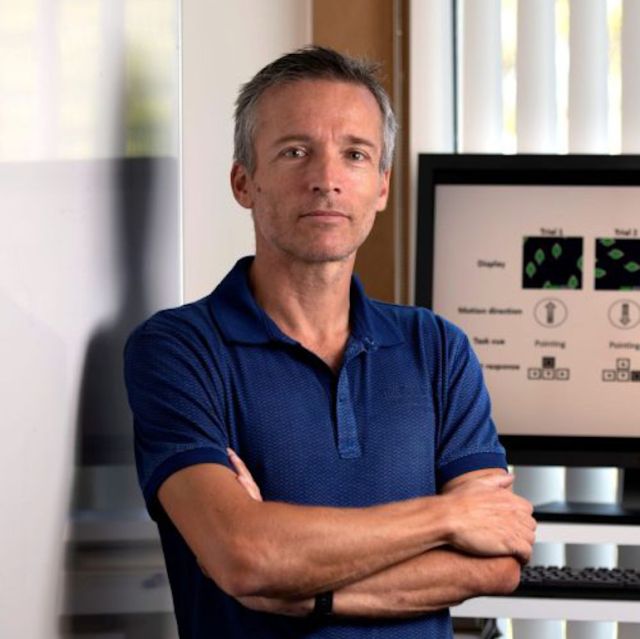
Mark Steyvers
Professor of Cognitive Sciences University of California, Irvine
"Mental Models in Human-AI Collaboration"
Abstract: Artificial intelligence (AI) and machine learning models are being increasingly deployed in real-world biomedical applications. In many of these applications, there is strong motivation to develop hybrid systems in which humans are assisted by AI algorithms, leveraging their complementary strengths and weaknesses. I will present research that investigates the cognitive decision process, and the mental models that people form of the AI in different paradigms for AI-assisted decision-making. In addition, I will also discuss how the AI can form mental models of the human decision-maker to optimize assistance. I will show some recent results on theory-of-mind experiments where the goal is for individuals and machine algorithms to predict the performance of other individuals in image classification and general knowledge tasks. The results show that humans generally outperform algorithms in mindreading tasks. I will discuss several research directions designed to close the gap.
Bio: Mark Steyvers is a Professor of Cognitive Science at UC Irvine and Chancellor’s Fellow. He has a joint appointment with the Computer Science department and is affiliated with the Center for Machine Learning and Intelligent Systems. Recently, in projects on human-AI collaboration, he has started to investigate how humans can collaborate with AI / Machine learning models to amplify and augment human decision-making
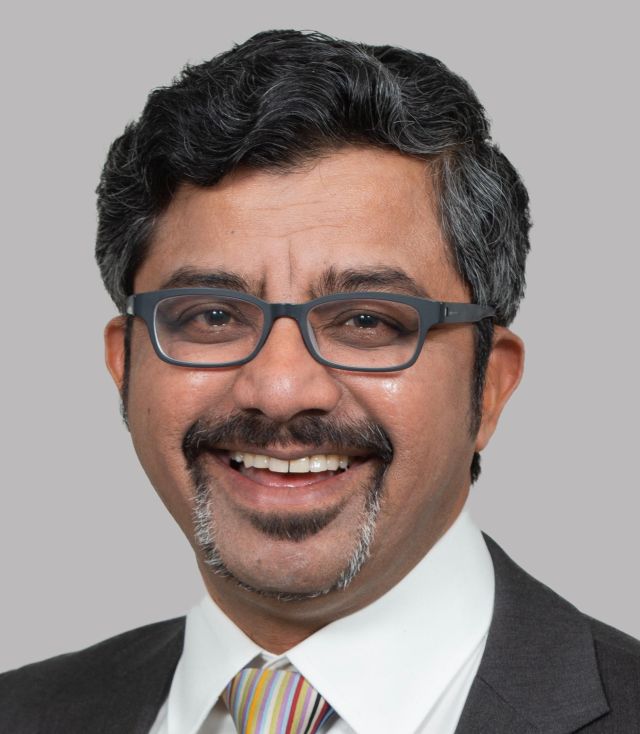
S. Shyam Sundar
Jimirro Professor of Media Effects
Penn State University
"Responsible AI: Enabling User Calibration of Trust with Interactive Interfaces"
Abstract: This talk will describe how the affordance of interactivity can help combat overtrust and undertrust in AI systems by enabling users to calibrate their trust. It will describe the speakers’ model of Human-AI Interaction (HAII), based on his Theory of Interactive Media Effects (TIME), which proposes two mechanisms—cues and actions—by which technological affordances shape user cognitions. It will explain how we can promote responsible AI by embedding warranted trustworthiness cues on the interface and providing interactive opportunities for users to enhance the transparency and explainability of automated systems.
Bio: S. Shyam Sundar is Jimirro Professor of Media Effects in the Bellisario College of Communications at Penn State University. He is the founder and current co-director of the Media Effects Research Laboratory. He also serves as director of the university-wide Center for Socially Responsible Artificial Intelligence. He edited the Handbook of the Psychology of Communication Technology (2015) and served as editor of the Journal of Computer-Mediated Communication (2013-2017).
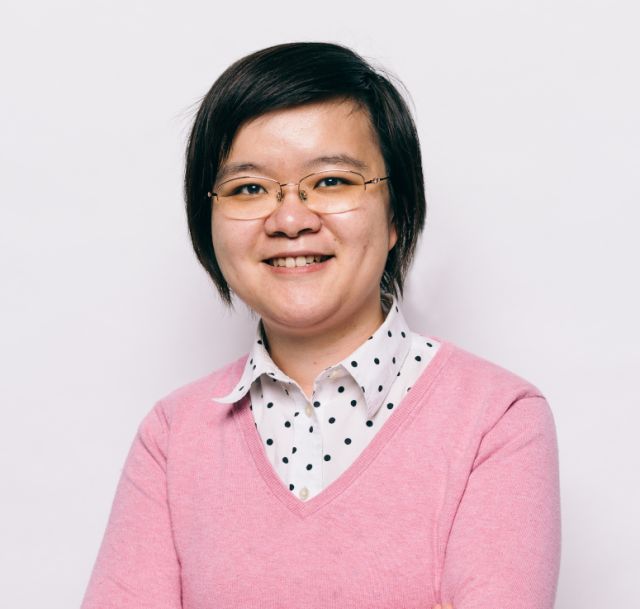
Ming Yin
Assistant Professor of Computer Science
Purdue University
"Towards a Science of Human-AI Decision Making: Empirical Understandings, Computational Models, and Intervention Designs"
Abstract: Artificial intelligence (AI) technologies have been increasingly integrated into human workflows. For example, the usage of AI-based decision aids in human decision-making processes has resulted in a new paradigm of human-AI decision making—that is, the AI-based decision aid provides a decision recommendation to the human decision makers, while humans make the final decision. The increasing prevalence of human-AI collaborative decision making highlights the need to understand how humans and AI collaborate with each other in these decision-making processes, and how to promote the effectiveness of these collaborations. In this talk, I'll discuss a few research projects that my group carries out on empirically understanding how humans trust the AI model via human-subject experiments, quantitatively modeling humans' adoption of AI recommendations, and designing interventions to influence the human-AI collaboration outcomes (e.g., improve human-AI joint decision-making performance).
Bio: Ming Yin is an Assistant Professor in the Department of Computer Science, Purdue University. Her current research interests include human-AI interaction, crowdsourcing and human computation, and computational social science. She completed her Ph.D. in Computer Science at Harvard University, and received her bachelor degree from Tsinghua University. Ming was the Conference Co-Chair of AAAI HCOMP 2022. Her work was recognized with best paper awards at CHI, CSCW, and HCOMP.
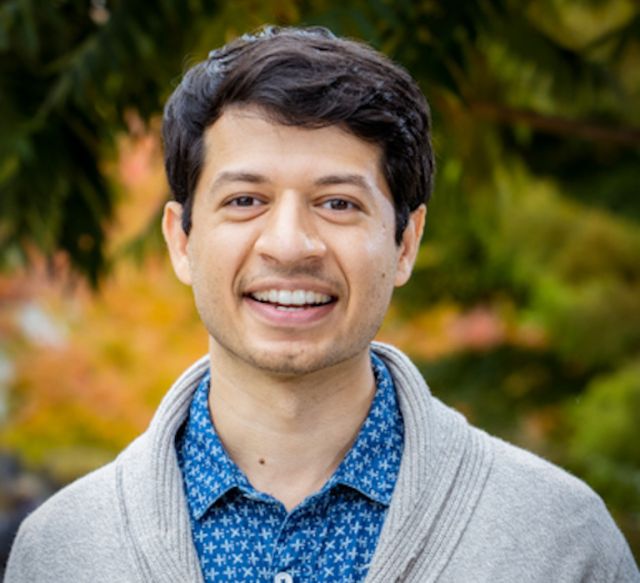
Gagan Bansal
Senior Researcher, Microsoft Research
"Understanding and Improving AI-Assisted Programming"
Abstract: AI code-recommendation systems (CodeRec), such as Copilot, can assist programmers inside an IDE by suggesting and autocompleting arbitrary code; potentially improving their productivity. To understand how these AI improve programmers in a coding session, we need to understand how they affect programmers' behavior. To make progress, we studied GitHub Copilot, and developed CUPS -- a taxonomy of 12 programmer activities common to AI code completion systems. We then conducted a study with 21 programmers who completed coding tasks and used our labeling tool to retrospectively label their sessions with CUPS. We analyze over 3000 label instances and visualize the results with timelines and state machines to profile programmer-CodeRec interaction. This reveals novel insights into the distribution and patterns of programmer behavior, as well as inefficiencies and time costs. Finally, we use these insights to inform future interventions to improve AI-assisted programming and human-AI interaction.
Bio: Gagan Bansal is a researcher at Microsoft Research, Redmond where he conducts interdisciplinary research on Artificial Intelligence and Human-Computer Interaction. His research broadly focuses on enabling human-AI interactions that help augment human performance. At Microsoft Research, he is currently part of the Human-AI eXperiences Team (HAX), where he helps lead innovation on human-centered AI and responsible AI. Prior to joining MSR in 2022, he graduated with a Ph.D in Computer Science from University of Washington, Seattle where he was advised by Dan Weld and was a part of the UW Lab for Human-AI Interaction.
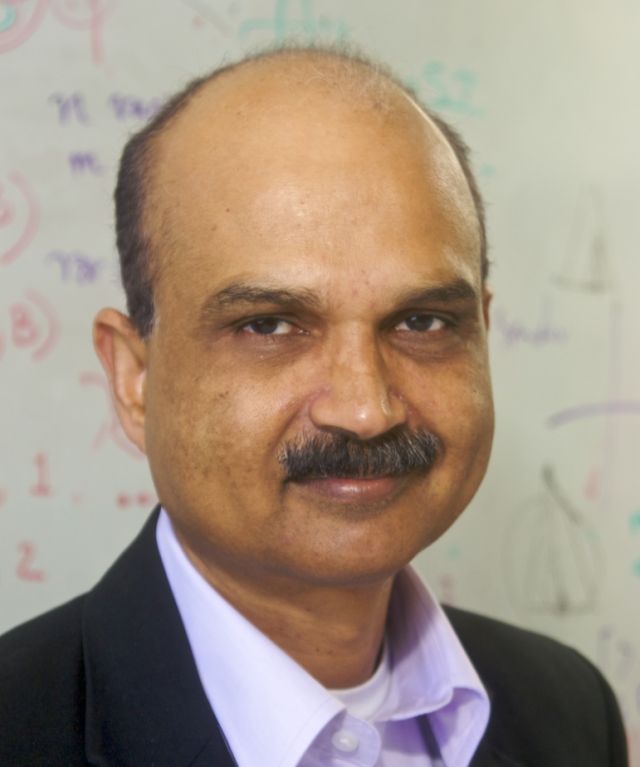
Ambuj Singh
Distinguished Professor of Computer Science and Biomolecular Science and Engineering
University of California, Santa Barbara
"Explaining Group Decision Processes Under Risk"
Abstract: We present a model for how a group’s decision process can be explained by the inherent risk-reward profile of constituent individuals and the group’s influence system. The model is validated empirically through human subject studies. We also present preliminary ideas on how “virtual” behaviors can be generated from a latent space and how the robustness of a group’s decision making can be analyzed.
Bio: Ambuj K. Singh is a Distinguished Professor of Computer Science at the University of California, Santa Barbara, with a part-time appointment in the Biomolecular Science and Engineering Program. He received a B.Tech. degree from the Indian Institute of Technology, Kharagpur, and a PhD degree from the University of Texas at Austin. His research interests are broadly in the areas of network science, machine learning, social networks, and bioinformatics. He has published 250 technical papers over his career. He has led a number of multidisciplinary projects including UCSB’s Information Network Academic Research Center funded by the Army, Interdisciplinary Graduate Education Research and Training (IGERT) program on Network Science funded by the NSF, and the Multidisciplinary University Research Initiative (MURI) on Network Science of Teams funded by the US Army. He has worked with over 50 graduate and postdoctoral students over his career, including over 30 PhD students.