Webinar: "Manipulation-Proof Machine Learning"
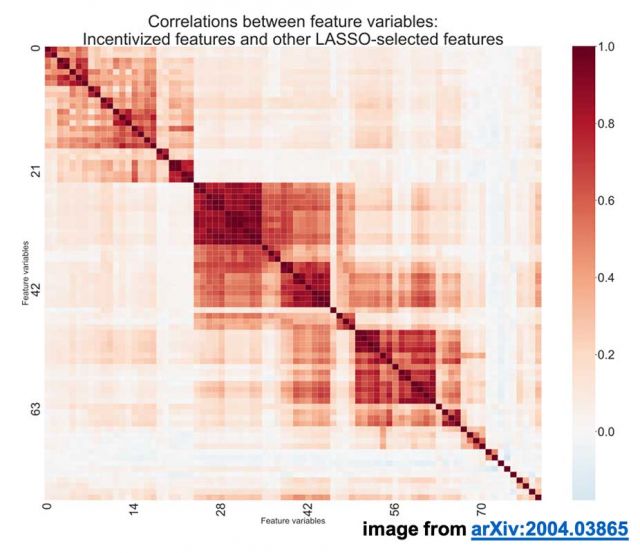
Daniel Björkegren, Brown University, "Manipulation-Proof Machine Learning"
Friday, April 2, 2021, 10:00am
To request the Zoom link, please contact Heather Royer
Host: Dick Startz, Department of Economics
Abstract: An increasing number of decisions are guided by machine learning algorithms. In many settings, from consumer credit to criminal justice, those decisions are made by applying an estimator to data on an individual's observed behavior. But when consequential decisions are encoded in rules, individuals may strategically alter their behavior to achieve desired outcomes. This paper develops a new class of estimator that is stable under manipulation, even when the decision rule is fully transparent. We explicitly model the costs of manipulating different behaviors, and identify decision rules that are stable in equilibrium. Through a large field experiment in Kenya, we show that decision rules estimated with our strategy-robust method outperform those based on standard supervised learning approaches.
Speaker Bio: Daniel Björkegren joined the PSTC and Brown University in 2014. He studies the process of economic development using new sources of data, including records generated by mobile phones. Björkegren's work has shown that patterns in mobile phone usage can be used to identify borrowers who will repay loans, making it possible to create credit scores for millions of households who do not have bank accounts. His current work in Rwanda uses operator records to reveal the network of value generated by mobile phones, and to simulate how the market would have evolved under different policies.